Publications
Research and Articles
My research addresses current AI and analytics ethics questions faced by organizations. I use data analytics, machine learning, qualitative interview-based research methodologies to study AI ethics from multiple angles.
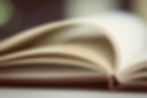
Removing Demographic Data Can Make AI Discrimination Worse
(Stephanie Kelley, Anton Ovchinnikov, Adrienne Heinrich, David R. Hardoon)
Harvard Business Review (online), 2023
A recent study suggests that denying AI decision makers access to sensitive data actually increases the risks of discriminatory outcome. That’s because the AI draws incomplete inferences from the data or partially substitutes by identifying proxies. Providing sensitive data would eliminate this problem, but it is problematic to do so in certain jurisdictions. The authors present work-arounds that may answer the problem in some countries.
Tailoring Explainable Artificial Intelligence: User Preferences and Profitability Implications for Firms
(Stephanie Kelley, Anton Ovchinnikov, Gabriel Ramolete, Keerthana Sureshbabu, Adrienne Heinrich)
Work in Progress, 2023
We conduct a lab-in-the-field experiment at a large institutional lender in Asia to study the preferences of real AI users (loan officers) with respect to the tailoring of explainable artificial intelligence (XAI). Our experiment utilizes a choice-based conjoint (CBC) survey in which we vary the XAI approach, the type of underlying AI model (developed by the lenders' data scientists with real data on the exact loans that our experimental subjects issue), the number of features in the visualization, the applicant aggregation level, and the lending outcome. We analyze the survey data using Hierarchical Bayes method, generating part-worth utilities for each AI user and at the sample level across every attribute combination. We observe that (i) the XAI approach is the most important factor, more than any other attribute, (ii) AI users prefer certain combinations of XAI approaches and models to be used together, (ii) user prefer nine or six features in the XAI visualizations, (iv) users do not have preferences for the applicant aggregation level, (v) their preferences do not change across positive or negative lending outcomes, and (vi) user preferences do not match the profitability rankings of the AI models. We then present a cost of misclassification profitability analysis across several simulated levels of AI user algorithm aversion. We show how firms can strategically combine models and XAI approaches to drive profitability; integrating the preferences of the AI users who are to incorporate AI models into their decision-making, with those of the data scientists who build such models.
Employee Perceptions of Effective AI Principle Adoption
(Stephanie Kelley)
Journal of Business Ethics, 2022
This study examines employee perceptions on the adoption of artificial intelligence (AI) principles in their organizations. 49 interviews were conducted with employees of 24 organizations across 11 countries. Participants worked directly with AI across a range of positions, from junior data scientist to Chief Analytics Officer. The study found that there are eleven components that could impact the effective adoption of AI principles in organizations: communication, management support, training, an ethics office(r), a reporting mechanism, enforcement, measurement, accompanying technical processes, a sufficient technical infrastructure, organizational structure, and an interdisciplinary approach. The components are discussed in the context of business code adoption theory. The findings offer a first step in understanding potential methods for effective AI principle adoption in organizations.
Anti-discrimination Laws, AI, and Gender Bias: A Case Study in Non-mortgage Fintech Lending
(Stephanie Kelley, Anton Ovchinnikov, David R. Hardoon, & Adrienne Heinrich)
Manufacturing & Service Operations Management, 2022
*Selected by IRCAI & UNESCO as a Global Top 100 AI Solutions for the UN SDGs
We study the impact of the existing anti-discrimination laws in different countries on gender bias in the non-mortgage consumer fintech lending setting. Building on the study of discrimination in operations, financial economics, and computer science, our paper investigates the impact and drivers of discrimination in machine learning models, trained on the alternative data used by fintech firms, and provide technically and legally permissible approaches for firms to reduce discrimination, whilst managing profitability.
A Code of Conduct for the Ethical Use of Artificial Intelligence in Financial Services
(Stephanie Kelley, Yuri Levin, & David Saunders)
2018
A public policy paper written in partnership with several large Canadian banks with principles for the ethical use of artificial intelligence in the Canadian financial services industry.